MoLiAIR
General context
Over time radon studies have involved a multidisciplinary range of applications from epidemiological studies targeting the carcinogenic impact of chronic exposure to radon in the residential or workplace buildings to Earth science studies in the frame of seismic and volcanic surveillance. Radon is the most important source of ionizing radiation of natural origin, being classified as a Group 1 carcinogen. According to the EC Directive, the Member States must promote actions to identify buildings with annual indoor radon concentration (AIRC) exceeding the reference level – RL (300 Bq/m3), but no clear measurement requirements are specified. Although it is not mandatory, an acceptance of the ISO 11665-8 (2019) standard is specific to laboratories that perform indoor radon measurements in European countries. According to this standard, a measurement period of at least two months is required.
A pertinent question is whether these two or, most often, three-month measurements may be representative for the AIRC, stipulated in the EC Directive and Radon Action Plan, respectively. Per se, the indoor radon concentrations can show a large temporal (diurnal, weekly and seasonal) variations. Although an increase in exposure duration leads to a reduction in uncertainties associated with temporal variations, even an exposure period of three months, depending on the season in which the measurement takes place, may be associated with uncertainties of up to 200%. A concession was made in the most radon survey by reporting semiannual or quarterly intervals on which temporal correction factors (TCF) were applied in order to estimate AIRC. However, many countries do not have such a database. For example, in Romania TCF developed for UK have been used for several campaigns.
Objectives
First objective of this proposal is to develop and implement a methodology to define the Most Likely Interval associated with AIRC (MLI-AIRC). Although is like the classical Confidence Interval defined in the inferential statistics, which means that the outcome is obtained with a certain probability, the MLI-AIRC refer to the information related to the measurement period, the duration of the measurement, the errors associated with the device used to perform radon measurements, as well as those variables that can affect the variability of radon measurements. If RL is within this interval, additional investigations are mandatory, otherwise, no further measurements are required. To define the MLI-AIRC, we will start from multiparametric statistical models that will consider the variability of the already defined correction factors (an easy approach for those countries that have such databases) follow by predictive models starting from a wide list of environmental and meteorological variables monitored at the same time with indoor radon to keep the most relevant ones in the final model.
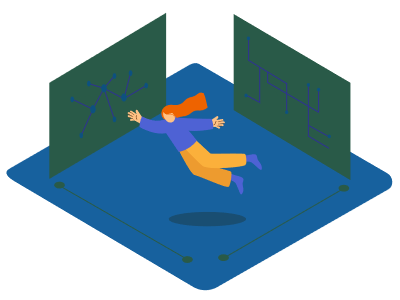
On the other hand, radon exposure at work is much more subtle and the 2013/59/Euratom Directive does not clearly specified if the measured annual radon concentration must be representative for the radon exposure during working time or not. An overestimation of the real exposure to radon in the case of daytime work and an underestimation for the nighttime work activities is expected following the use of integrated measurement methods for radon concentration assessment, as pointed out by Bochicchio . In addition, the 2013/59/Euratom Directive does not stipulate if work-specific temporal correction factors or those specific to the residential buildings should be applied. Therefore, it is very important to have access to complex data in terms of radon concentrations and exposure timeframe in different places (residential and workplaces) and to combine these data to generate a time-weighted estimates of radon exposure. Therefore, we propose the first temporal correction factors database established for radon exposure in workplaces as the second objective of the project. The study will involve both continuous and integrated measurements during one year at workplaces. Similar measurements will be performed in residential buildings, at the same time. In this way, we will have the necessary evidence for the further application of residential TCF to the workplace buildings or a new methodology that involves the use of custom correction factors depending on the type and destination of the building. In addition, through the measurements performed at the same time by the two methods (continuous and integrated), we will be able to evaluate the feasibility of using active radon measurements as an alternative to the “golden standard” represented by the integrated method.

It is well known that geology is responsible for radon level in the soil gas and, implicitly, in indoors. A thorough analysis of the contribution of variations in soil properties around the building (humidity and temperature), along with continuous monitoring of radon in the soil could provide new evidence of the impact on seasonal variability of radon inside buildings. A continuous monitoring of radon in the soil gas represents an important and active research topic in the field of Earth science, bringing new arguments for treating radon as a precursor to earthquakes. Thus, the presence of anomalies in radon temporal variations identified by various algorithms (i.e., the difference between the measured value and the predicted one) could be correlated with the tectonic movements due to earthquakes. However, a major step in this direction is the ability to distinguish between anomalies induced by these tectonic movements and those due to environmental factors, which can also impact the temporal variations of indoor radon.
Therefore, the prediction analysis can have a double role, allowing the identification of the contribution on TCF, as well as the elaboration of algorithms for the identification of anomalies useful in seismology. While mechanistical models require all possible parameters to be determined, statistical and ML models are based on data collected on the targeted parameters and on predictors. Predictions can be used to take right decision to explain the source of detected anomalies. Anomalies can be explained as sensor errors or can be corelated with change of occupancy behavior or building degradation. Analysis of multivariate time series can be used to cluster and classify different buildings based on their occupancy, type of materials used, height, and geolocation. Clustering can help to increase the accuracy of predictive models trained for different type of buildings. The current project represents a follow-up of the POC SmartRadEn project (Contract No. 22/01.09.2016, ID P_37_229, MySmis code: 103427). Unlike the previous project which aimed at the monitoring of IAQ in 100 buildings and remediation for 10 houses, alongside the development of the ICA device, in this project the use of the infrastructure (ICA) for the definition of MLI-AIRC is followed, respectively the performance of continuous radon measurements in the soil gas.